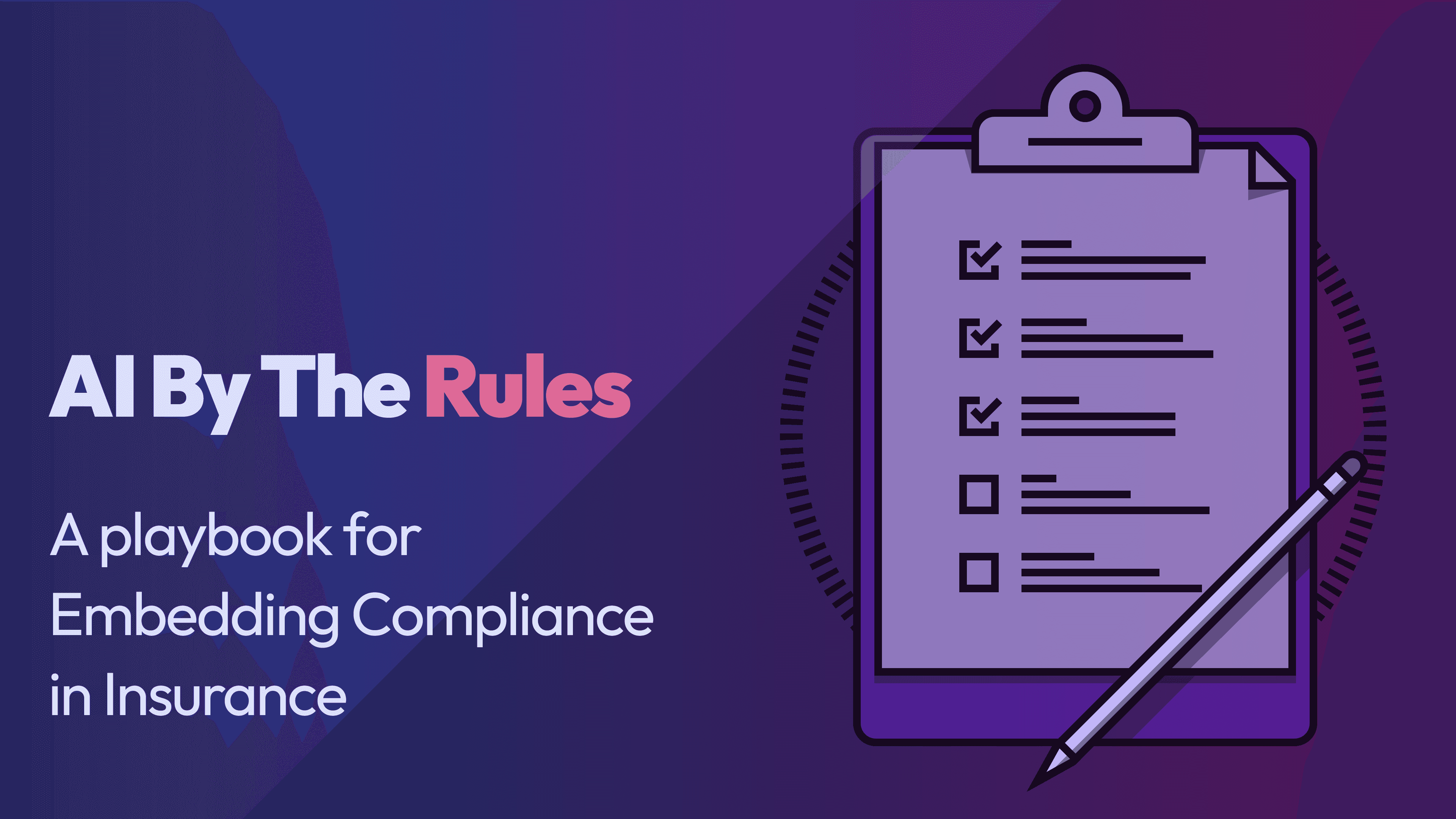
AI By the Rules: A playbook for Embedding Compliance in Insurance
Compliant AI Playbook for Insurers - Part 3
Introduction
In our ongoing series on AI compliance and ethical implementation, recent discussions have identified critical pitfalls and compliance concerns associated with the integration of AI across various sectors. Artificial Intelligence (AI) holds the potential to transform the insurance industry; however, it also presents significant regulatory challenges. Establishing compliance within AI frameworks is essential to ensure the development of ethical, legal, and trustworthy AI systems.
This guide delineates six key steps for embedding compliance in AI, emphasizing practical implementation strategies. These strategies are informed by the compliance compass presented in our previous instalment. By addressing both the challenges and opportunities within this rapidly evolving landscape, we aim to provide valuable insights that empower organizations to navigate the complexities of AI integration effectively.
Step 1:
Establish Clear Ethical and Compliance Guidelines
Embedding compliance in AI necessitates establishing clear ethical and compliance guidelines. It is essential to identify and delineate pertinent regulations, such as the California Consumer Privacy Act (CCPA) and the General Data Protection Regulation (GDPR), as these form the cornerstone of compliance within AI-driven processes in the insurance industry. This foundational framework will be explored in detail in the concluding segment of this series, highlighting both the challenges and opportunities that lie ahead in this rapidly evolving field (California State Legislature, 2018 ; European Union, 2016)
Privacy and Data Protection
It is imperative to ensure that personal data is managed in accordance with regulations such as GDPR and CCPA, safeguarding individuals' rights and privacy.
Bias Mitigation
Implementing proactive strategies to identify and reduce biases within AI models is crucial for fostering fairness and equity in AI-driven outcomes.
Accountability
Establishing clear roles and responsibilities is vital, including the formation of an audit team dedicated to overseeing compliance efforts and ensuring adherence to established standards.
By focusing on these components, organizations can build a strong foundation for compliance that not only meets regulatory standards but also showcases their commitment to ethical AI practices, making them more appealing to users exploring AI products.
Example
A visual representation of a compliance framework (refer to Figure 1) effectively illustrates the integration of critical elements throughout the AI lifecycle. This chart serves as a valuable tool for visualizing the continuous loop of compliance checks essential from the design phase to deployment.
Figure 1. Compliance Framework for AI in Insurance
This figure delineates a cyclical process in which compliance is consistently revisited and updated to ensure that AI systems remain aligned with ethical standards and adapt to evolving regulations. This approach not only addresses current industry challenges but also underscores the importance of proactive compliance in fostering trust and accountability within the AI landscape.
Step 2:
Incorporate Compliance in AI Design and Development
Compliance by Design: Establishing Ethical Foundations
Integrating compliance into AI models from the design phase is critical for ensuring these systems operate with integrity. The "Compliance by Design" framework embeds fundamental principles such as privacy, fairness, and transparency directly into the architecture of AI systems. This methodology is in alignment with international standards, including those set forth by the European Union, which advocate for responsible AI development (European Union, 2016).
Harmonizing AI Capabilities with Human Oversight
In the insurance sector, where AI-driven decisions can significantly impact customers’ lives, achieving a balance between the advanced predictive capabilities of AI and necessary human oversight is paramount. A hybrid approach, in which AI recommendations undergo scrutiny by human experts, mitigates the risks associated with excessive reliance on predictive models. This collaboration between AI and human judgment enhances the accuracy and fairness of decisions, ensuring that AI outputs are critically evaluated to prevent errors stemming from incomplete or biased data (Daugherty & Wilson, 2018).
Addressing Over-Reliance on Predictive Approaches
In the rapidly evolving landscape of AI, it is imperative to avoid undue dependence on predictive models, which can lead to substantial errors and biases. By integrating predictive analytics with human oversight, organizations can improve both the accuracy of their decisions and the fairness of outcomes. This dual approach capitalizes on the strengths of AI technology while ensuring that human expertise is applied to critically assess AI-generated recommendations. Such a strategy not only addresses current challenges but also unlocks new opportunities for enhanced decision-making within the insurance industry (Dietvorst, Simmons, & Massey, 2015).
Example
Consider a scenario in an insurance company where an AI model is developed to assess disability claims. By adopting the "Compliance by Design" framework, the team integrates privacy protocols from the initial design stages, ensuring that personally identifiable information (PII) is anonymized and protected in accordance with GDPR requirements.
Additionally, the model includes features that actively check for bias in disability claim decisions. For example, the AI is programmed to flag inconsistencies in claim approvals based on factors like age, gender, or geographic location, which may indicate underlying biases. Human experts regularly review these flagged decisions to provide oversight and ensure the fairness of the AI’s conclusions.
This compliance-centric development process enhances trust in the AI system and guarantees equitable outcomes, ultimately fostering a stronger relationship between the insurance provider and its clients. Such careful integration of ethical considerations into the AI lifecycle showcases how organizations can bolster their compliance efforts while maintaining the integrity of their AI applications in the disability insurance space.
Compliance Strategy | Implementation | Outcome |
---|---|---|
Privacy Protocols | Integration of GDPR/CCPA-compliant anonymization and data protection measures from the initial design phase. | Ensures that Personally Identifiable Information (PII) is secure and compliant with regulations. |
Bias Detection Features | AI model programmed to flag inconsistencies in claim approvals based on factors like age, gender, or geographic location. | Identifies potential biases, ensuring that decisions are fair and unbiased. |
Human Oversight | Regular review of AI-flagged decisions by human experts to validate and ensure fairness. | Enhances the accuracy and fairness of AI decisions, building trust in the AI system. |
Compliance by Design | Adoption of a framework that embeds privacy, bias detection, and human oversight throughout the AI development process. | Promotes ethical AI practices and strengthens the relationship between the insurance provider and clients. |
Table 1: Compliance-Centric Development in AI Disability Claims Assessment, summarizing the compliance strategies, their implementation in the AI model, and the outcomes, clearly showing how the example aligns with a compliance-centric approach to AI development in the insurance industry.
Step 3:
Adopt Explainable AI (XAI) and Transparency Practices
Transparency is crucial for building trust in AI-driven decision-making processes. Implementing Explainable AI (XAI) techniques ensures that AI decision-making is both transparent and interpretable (Selbst & Barocas, 2018).
XAI Integration:
To enhance understanding, incorporate tools that offer visual and textual explanations of AI decisions. These tools can help users grasp how AI arrives at specific conclusions, enabling them to challenge or validate outcomes effectively. By presenting complex data in an accessible format, stakeholders can engage more meaningfully with AI systems.
Model Documentation:
It's essential to maintain comprehensive documentation of AI models and their decision-making pathways. This documentation should include details on data sources, algorithm choices, and the reasoning behind specific model configurations. By doing so, organizations can foster accountability, allowing for better audits and reviews of AI processes. Regular updates to this documentation can help ensure that it reflects any changes made to models or their underlying assumptions.
Benefits of XAI and Transparency
By prioritizing transparency through XAI integration and thorough documentation, organizations can create a more trustworthy and understandable AI landscape, ultimately benefiting users and stakeholders alike.
Example
Consider an insurance company that implements XAI to explain how claims are evaluated. The system provides clear, understandable insights into the factors influencing claim approvals or rejections. This transparency not only builds trust with customers but also allows the company to audit its processes more effectively, ensuring compliance with ethical standards and regulatory requirements.
Step 4:
Implement Data Governance and Bias Mitigation
In the insurance industry, effective data governance is not just a regulatory requirement; it's the foundation for building trustworthy AI systems that align with ethical standards. This involves instituting stringent quality control measures, including continuous monitoring and validation of the data used in AI models. By developing tailored data governance frameworks, insurance companies can ensure that their AI systems are transparent, accountable, and free from biases that could lead to discriminatory practices or skewed decision-making.
Bias Audits and Ethical Oversight
Conducting regular bias audits is crucial for identifying and addressing potential biases within AI models. In the insurance sector, this means leveraging diverse and representative datasets that reflect the varied demographics of policyholders. These audits should be complemented by the establishment of an ethics oversight committee, which plays a key role in guiding data governance practices. This committee should consist of cross-functional experts, including legal, ethical, and technical professionals, to ensure a holistic approach to AI ethics and compliance. Their continuous oversight helps maintain transparency and trust, critical in an industry where fairness and equity are paramount.
By focusing on these tailored strategies, insurance companies can not only mitigate the risks associated with bias and ethics in AI but also harness these technologies to enhance the fairness and accuracy of underwriting, claims processing, and customer service.
Accountability Mechanisms
Establishing robust accountability mechanisms is essential to maintaining compliance and fostering a culture of responsibility within AI operations. This includes setting up independent oversight committees, conducting rigorous compliance checks, and clearly defining the responsibilities of both AI developers and users. For instance, implementing specific guidelines to prevent misuse or ethical violations is crucial in the insurance industry, where the consequences of non-compliance can be particularly severe(Barocas, Hardt, & Narayanan, 2019).
Measure | Description | Frequency |
---|---|---|
Independent Oversight | Form committees to monitor AI development and deployment, focusing on insurance-specific regulations. | Quarterly |
Compliance Checks | Conduct regular assessments to ensure AI processes meet industry standards and regulatory requirements. | Monthly |
Policy Enforcement | Implement and enforce clear consequences for ethical violations, tailored to insurance industry risks. | As Needed |
Table 2. Accountability Measures in AI Compliance: outlining a structured approach to implementing accountability measures within the insurance industry
Example of Bias in AI
In recent years, several insurance companies have come under scrutiny for using AI algorithms that unintentionally perpetuate bias. For instance, some models have been found to discriminate against certain demographic groups when assessing risk and determining premiums. These biases often stem from historical data that reflect systemic inequalities, such as lower income levels or reduced access to healthcare in specific communities. As a result, individuals from these groups may face higher premiums or denial of coverage, exacerbating existing disparities. Recognizing the ethical implications, some AI developers for insurance providers are now working to audit their algorithms and incorporate more diverse data sets to ensure fairness and equity in their pricing and underwriting practices. This highlights the critical need for ongoing bias assessment and the inclusion of diverse perspectives in the development of AI systems within the insurance sector.
Step 5:
Train, Engage, and Adapt
Collaborative Training for AI Developers and Users
AI developers and users occupy distinct yet complementary roles in the deployment of AI systems. Tailored training programs are essential for both demographics to ensure efficacy. Developers must grasp the ethical frameworks, compliance standards, and technical specifications necessary for constructing responsible AI systems. Concurrently, users require training to utilize AI tools effectively while remaining cognizant of potential ethical and compliance issues. Regular, collaborative training sessions uniting developers and users can cultivate a profound understanding of each other’s responsibilities, thereby enhancing collaborative efforts.
Continuous Monitoring and Adaptive Training
Ongoing monitoring of AI systems for compliance and ethical adherence is imperative. The insights gleaned from these assessments should inform continuous training and updates. When new challenges or regulatory shifts are identified, it is crucial to promptly adapt training programs to address these emerging developments. This dynamic approach ensures that both AI developers and users stay informed about current compliance standards, ethical principles, and best practices. Establishing regular feedback loops, wherein monitoring results are evaluated and training is updated accordingly, can significantly enhance the relevance and effectiveness of AI practices.
Engaging Stakeholders in the Compliance Process
The active engagement of stakeholders throughout the AI lifecycle is vital for upholding compliance and ethical standards. The creation of forums, workshops, or advisory panels where stakeholders—including AI developers, users, regulators, and customers—can engage in discussions about AI development and compliance cultivates a culture of transparency and accountability. Such platforms empower stakeholders to express concerns, share insights, and collaborate on solutions, ultimately leading to more robust and compliant AI systems.
Example
Consider an insurance company that systematically monitors its AI systems for adherence to evolving regulations and ethical standards. Upon detecting a new bias in the claims processing AI, this discovery initiates an update to the training curriculum for both developers and users. A collaborative workshop is convened to discuss these findings, engaging developers, users, and key stakeholders. This session not only communicates the new compliance requirements but also solicits valuable input from stakeholders on system enhancements. This adaptive, collaborative methodology ensures the company remains at the forefront of ethical AI practices, continuously refining both the technology and the competencies of those involved in its development and application.
This strategy of ongoing, collaborative training and stakeholder engagement guarantees that AI systems uphold compliance and ethical standards, even as industry norms and regulations evolve Greene et al. (2019).
Step 6:
Establish Monitoring, Auditing, and Reporting Mechanisms
Continuous monitoring and regular auditing are essential for ensuring the long-term compliance of AI systems. By adopting a structured approach, organizations can proactively identify and mitigate potential ethical issues. The establishment of comprehensive reporting mechanisms is crucial for maintaining alignment with ethical standards and regulatory requirements, as emphasized by Raji et al. (2020). These mechanisms should encompass routine assessments of AI performance, bias evaluations, and adherence to privacy regulations.
Example
A timeline graph (refer to Figure 2) effectively illustrates the structured approach to continuous monitoring within AI systems. This timeline should delineate specific intervals for comprehensive evaluations, including monthly assessments for algorithmic fairness, quarterly reviews of compliance with data protection laws, and annual audits to align with evolving ethical guidelines.
Figure 2. Continuous Monitoring Timeline for Ethical AI Compliance
This figure delineates the continuous monitoring process in AI systems, showcasing critical milestones for ethical assessments and necessary updates. It serves as a strategic roadmap for organizations, ensuring that their AI applications not only comply with existing standards but also adapt to emerging challenges and ethical considerations. By adhering to this timeline, organizations can cultivate greater trust in their AI systems and foster a culture of accountability and transparency.
Conclusion
In an era marked by rapid advancements in artificial intelligence, the importance of ethical compliance and transparency cannot be overstated. This article has illuminated the critical steps organizations must take to integrate responsible AI practices, from establishing comprehensive monitoring frameworks to fostering collaborative training environments for developers and users. By prioritizing ethical standards and stakeholder engagement throughout the AI lifecycle, organizations not only cultivate trust and accountability but also enhance the efficacy of their AI systems. Emphasizing continuous adaptation and responsive training programs will ensure that stakeholders remain informed and engaged as the regulatory landscape evolves. Ultimately, fostering a culture of transparency and responsibility in AI development is essential for its sustainable growth and broader societal acceptance. As organizations navigate the complexities of ethical AI, they must commit to creating systems that are not only innovative but also align with the principles of fairness, respect, and accountability.
References
- California Legislative Information. (2018). California Consumer Privacy Act (CCPA). Retrieved from https://leginfo.legislature.ca.gov
- European Union. (2016). General Data Protection Regulation (GDPR). Retrieved from https://eur-lex.europa.eu/legal-content/EN/TXT/?uri=CELEX%3A32016R0679
- Daugherty, P. R., & Wilson, H. J. (2018). Human + Machine: Reimagining Work in the Age of AI. Boston: Harvard Business Review Press.
- Dietvorst, B. J., Simmons, J. P., & Massey, C. (2015). Algorithm aversion: People erroneously avoid algorithms after seeing them err. _Journal of Experimental Psychology: _General, 144(1), 114–126. https://doi.org/10.1037/xge0000033
- Barocas, S., Hardt, M., & Narayanan, A. (2019). _Fairness and machine learning. _Cambridge: MIT Press.
- Selbst, A. D., & Barocas, S. (2018). The intuitive appeal of explainable machines. Fordham Law Review, 87(3), 1085-1139.
- Raji, I. D., Bender, E. M., L. P. Ferryman, K., & Paullada, A. (2020). AI and the Everything in the Whole Wide World Benchmark: Reassessing Bias in AI. In Proceedings of the 2020 Conference on Fairness, Accountability, and Transparency (pp. 322–330).
- Greene, D., Hoffmann, A. L., & Stark, L. (2019). Better, nicer, clearer, fairer: A critical assessment of the movement for ethical artificial intelligence and machine learning. Proceedings of the Conference on Fairness, Accountability, and Transparency, 212–223.
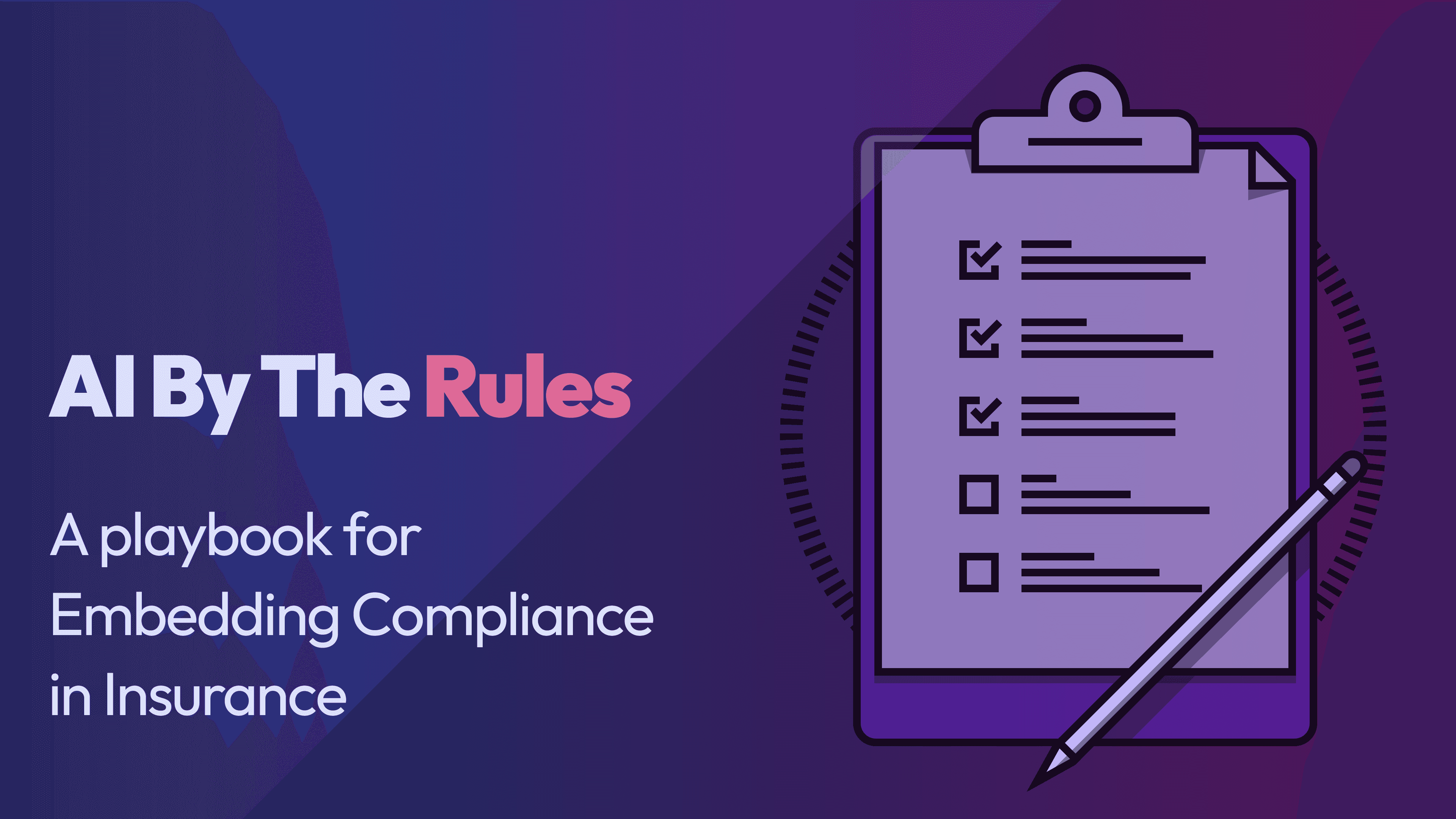