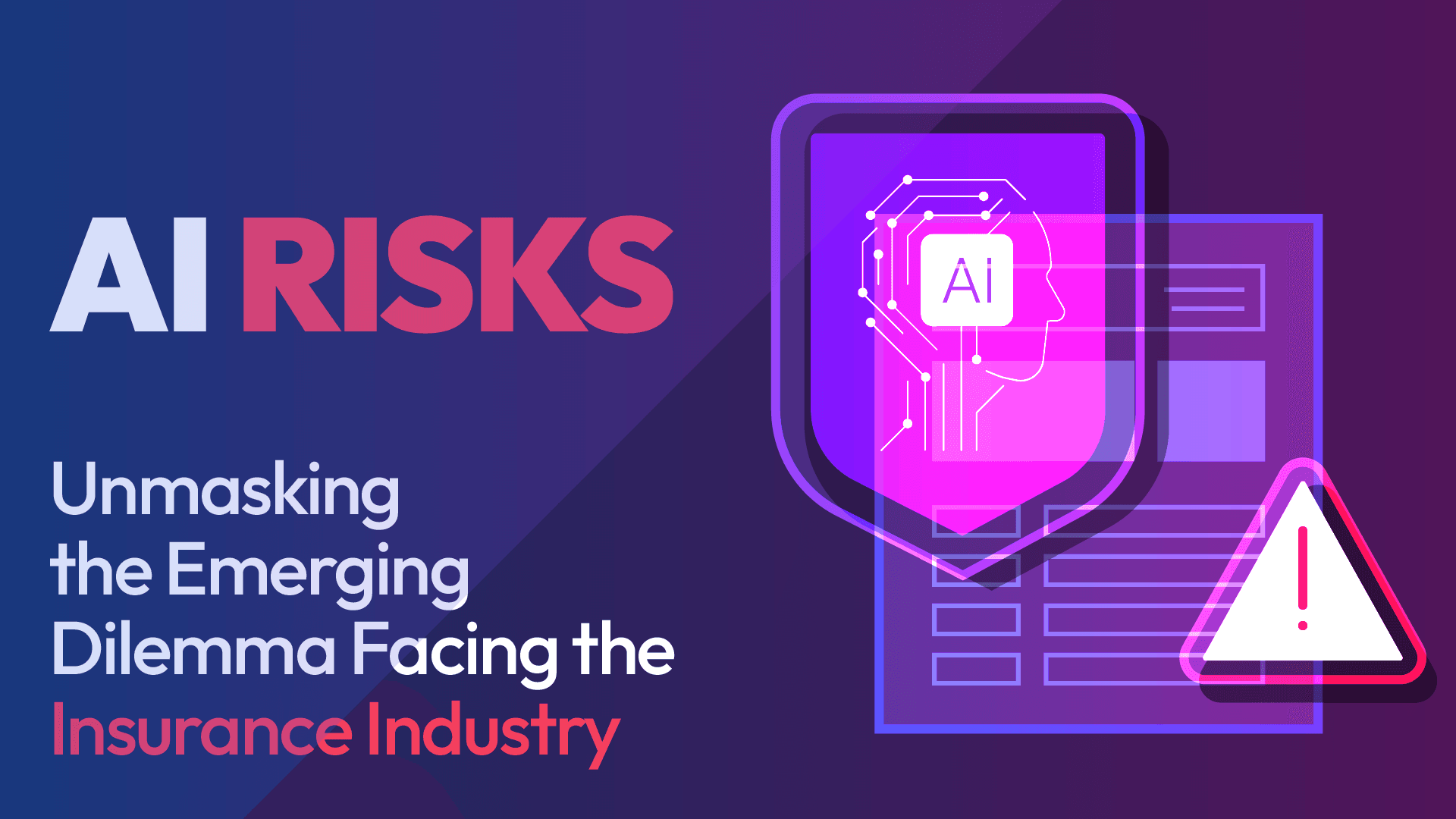
The Emerging Dilemma: Identifying the Compliance Risks in AI for Insurance
Introduction
As AI technology continues to advance at an astonishing pace, it reshapes industries and daily interactions in profound ways. Yet, with this progress comes a responsibility to address the ethical considerations that arise from AI implementation. Organizations must not only embrace innovation but also scrutinize the societal ramifications of their AI practices. This document delineates prominent pitfalls frequently encountered in AI integration, emphasizing the importance of adopting forward-thinking strategies to mitigate risks. By promoting an environment of transparency, inclusivity, and accountability, we can harness the full potential of AI while safeguarding the rights and privacy of individuals.
Data Privacy
Privacy Concerns and Challenges in Safeguarding Client Information
Privacy is an essential human right, empowering individuals with control over their personal data and its utilization. With AI systems processing extensive volumes of sensitive personal information, significant privacy concerns have arisen. Regulations such as the CCPA and GDPR establish stringent guidelines aimed at safeguarding consumer data, highlighting the necessity for explicit consent and transparency in data handling practices (European Union, 2016; California Legislative Information, 2018).
Nevertheless, AI systems inherently rely on the analysis of large datasets, including private information, to learn and enhance their functionalities. Consequently, the volume of personal data collected by these systems continues to increase, amplifying concerns regarding privacy and data protection. The proliferation of generative AI tools, including ChatGPT and Stable Diffusion, raises critical questions about the usage of our data—comprising articles, images, videos, and more—often without our explicit consent. As Mark McCreary, co-chair of the privacy and data security practice at Fox Rothschild LLP, articulated to CNN, “The privacy considerations with something like ChatGPT cannot be overstated. It’s like a black box.” When users input data into these platforms, McCreary notes, “You don’t know how it’s then going to be used.” (McCreary,2023)
Addressing these challenges is paramount as we navigate the complexities of AI and privacy. It is essential for stakeholders in the industry to advocate for robust data protection measures and to explore innovative solutions that align with both technological advancements and the safeguarding of individual rights.
This underscores the necessity for stringent data protection measures and ethical guidelines to govern the use of AI technologies.
Year | GDPR Comp. Rate | CCPA Comp. Rate | Notes |
---|---|---|---|
2016 | 50% | N/A | GDPR was still new, and CCPA had not been enacted. |
2018 | 65% | 40% | Both GDPR and CCPA regulations were becoming more enforced. |
2019 | 70% | 50% | Increased awareness and implementation of compliance measures. |
2020 | 75% | 60% | Enhanced compliance due to stricter enforcement and fines. |
2021 | 80% | 70% | Companies increasingly adopting comprehensive data protection strategies. |
2022 | 85% | 75% | Significant improvements in compliance through AI and machine learning applications. |
2023 | 90% | 80% | Continued emphasis on data privacy and security in AI systems. |
Table 1: Compliance rates of the insurance industry with GDPR and CCPA from 2016 to 2023.
Data Protection
Security Risks of Using AI with Sensitive Data
Organizations and companies that harness AI technology have a responsibility to proactively protect individuals' Personally Identifiable Information (PII) and mitigate the security risks associated with large language models (LLMs). The deployment of LLMs in various AI applications introduces significant security vulnerabilities, stemming from their capacity to generate and manipulate extensive data sets. Key risks include data breaches, unauthorized access, and the potential exploitation of sensitive information (BrightSec, 2023).
To safeguard data effectively, it is imperative to implement rigorous data security protocols, such as advanced encryption, anonymization techniques, and stringent access controls. Aligning data usage with its intended purpose and designing AI systems grounded in ethical principles are critical strategies to diminish these risks. Neglecting to enforce these measures can result in data breaches, leading to considerable financial repercussions and reputational harm for organizations (BrightSec, 2023).
By adopting a proactive approach and prioritizing data protection, companies can not only shield themselves from potential threats but also position themselves as leaders in a rapidly evolving technological landscape.
Dangerous Recommendations
The Risks and Probability of Harmful AI Outcomes
AI systems, particularly within the insurance sector, can produce dangerously misleading recommendations if not meticulously designed, implemented, and monitored. These advanced algorithms, while aimed at optimizing operations and enhancing decision-making, can erroneously classify legitimate transactions as fraudulent (resulting in false positives) or, conversely, overlook genuine fraudulent activities (leading to false negatives). Such inaccuracies can yield significant repercussions, including substantial financial losses for organizations and dissatisfaction among customers who may perceive themselves as unfairly treated or misjudged.
To mitigate these risks, it is imperative to establish rigorous protocols for continuous monitoring, validation, and updating of AI models. This ongoing oversight is crucial to ensure that algorithms remain effective and reliable, adapting to evolving behavioral patterns and emerging threats in the dynamic landscape of insurance fraud. By investing in comprehensive training and development processes for these systems, companies can enhance the accuracy of AI recommendations, thereby improving both operational efficiency and customer trust.
Addressing the challenges posed by AI in insurance not only highlights potential pitfalls but also underscores the opportunities for innovation and improvement within the industry. Embracing this forward-thinking approach can position organizations as leaders in leveraging technology to tackle industry challenges and optimize outcomes.
Confabulation
The creation of plausible yet inaccurate explanations by AI systems
Confabulation is a critical phenomenon in AI systems, where they produce plausible yet incorrect explanations for their decisions. This can create a misleading sense of understanding among users, ultimately undermining trust in these technologies. Stakeholders often face challenges in assessing the validity of AI outputs, which becomes increasingly important as AI integrates into various industries. The implications of confabulation extend to crucial areas such as healthcare and financial forecasting, making it imperative to address this issue.
To mitigate the risks associated with confabulation, Explainable AI (XAI) techniques are essential. These methodologies prioritize transparency and comprehensibility in AI decision-making processes, enabling users to grasp how AI reaches its conclusions. By implementing XAI, stakeholders can better analyze the rationale behind AI outputs, fostering trust and empowering users to make informed decisions based on AI guidance. As highlighted by Selbst & Barocas (2018), clarity in AI operations is vital for promoting reliability and accountability in increasingly technology-driven environments.
By addressing both the challenges of confabulation and the opportunities presented by XAI, we can enhance the efficacy of AI systems, ensuring they serve as reliable tools in navigating complex industry landscapes.
Intellectual Property
The protection of innovations and proprietary data against misuse or theft
Protecting AI innovations and proprietary data is absolutely crucial in today's digital landscape to prevent misuse, data leaks, and unauthorized access. Companies need to implement robust intellectual property (IP) protection strategies tailored to the intricate nature of AI technology. This involves not only securing patents and trademarks but also developing comprehensive policies that govern data usage and employee training on data security practices. Ensuring compliance with relevant laws and regulations, such as GDPR and CCPA, is vital for safeguarding technological advancements while maintaining a competitive edge in the market.
Furthermore, large language models (LLMs) present unique security risks that organizations must address proactively. One significant threat is intellectual property theft through model inversion attacks, where malicious actors may extract sensitive training data by reversing the model's outputs. This can lead to the exposure of proprietary information and trade secrets, potentially harming a company's reputation and financial standing (BrightSec, 2023).
Therefore, addressing these risks requires continuous monitoring and updating of security measures. Companies should invest in advanced cybersecurity technologies and practices, conduct regular audits, and train staff to recognize potential threats. Additionally, fostering a culture of security awareness across all levels of the organization is essential for protecting against evolving threats and ensuring the integrity of AI innovations.
Bias and Homogenization
The Influence of Historical Biases in AI Training Data on Decision-Making Outcomes
AI models trained on historical data frequently generate biased outcomes, which can significantly impact the fairness and equity of critical decisions made in various sectors, including hiring, lending, and law enforcement. Addressing these biases is crucial for ensuring that AI systems operate justly and effectively. This requires the implementation of comprehensive guidelines that advocate for diverse and representative training data, regular and thorough audits to assess bias levels, and continuous updates to the models to reflect changing societal norms and values (Mittelstadt et al., 2016; OWL.co, 2023).
Moreover, large language models (LLMs) can inadvertently reinforce existing biases embedded in their training data, which can perpetuate stereotypes and discrimination in generated content. This situation underscores the urgent need for robust bias mitigation strategies and ethical guidelines during the development and deployment of AI technologies. Such measures not only enhance the reliability of AI systems but also foster trust among users and stakeholders, ensuring that these powerful tools contribute positively to society (BrightSec, 2023).
Component Integration
The intricate process of integrating various AI components is crucial for
developing effective and reliable systems. Integrating various AI components—such as diverse data sources, sophisticated algorithms, and intricate decision-making processes—can pose significant challenges that demand careful consideration and expertise. The complexity of these integrations requires not only technical proficiency but also a deep understanding of how these components interact within the larger AI framework. Ensuring seamless and secure integration is crucial for the effectiveness and reliability of AI systems, as any hiccups in this process can have far-reaching consequences.
For instance, poor integration can lead to system failures that disrupt operations, data breaches that compromise sensitive information, and inaccurate fraud detection that undermines trust in the system's capabilities. Moreover, the implications of these failures can extend beyond immediate operational setbacks, potentially resulting in financial losses and damage to an organization’s reputation.
To mitigate these risks, comprehensive testing, validation, and continuous monitoring are essential to address integration challenges effectively. This involves regularly assessing the performance of integrated components, ensuring compliance with security protocols, and adapting to new data inputs or algorithmic developments. By prioritizing these practices, organizations can enhance the smooth functioning of their AI systems and ultimately harness their full potential in driving innovation and efficiency (NIST, 2021).
Conclusion
Organizations and companies leveraging AI must prioritize privacy and ethical considerations in the design and implementation of their systems. This involves maintaining transparency around data collection and usage, ensuring robust data security, routinely auditing for bias and discrimination, and developing AI systems aligned with ethical principles. By prioritizing these factors, companies can foster trust with their customers, mitigate reputational risks, and enhance relationships with stakeholders.
As AI technology becomes increasingly integrated into our daily lives, the importance of strong privacy and ethical considerations cannot be overstated. By committing to transparency, stringent data protection, and ethical design in AI systems, companies not only comply with regulatory standards but also cultivate enduring trust and positive relationships with customers and stakeholders. Responsible development and deployment of AI technologies will enable us to harness their benefits while safeguarding individual privacy and rights.
References
- BrightSec. (2023). Exploring the Security Risks of Using Large Language Models. Retrieved from https://brightsec.com/whitepapers/exploring-the-security-risks-of-using-large-language-models/
- California Legislative Information. (2018). California Consumer Privacy Act (CCPA). Retrieved from https://leginfo.legislature.ca.gov
- European Union. (2016). General Data Protection Regulation (GDPR). Official Journal of the European Union. Retrieved from https://eur-lex.europa.eu
- McCreary, M. (2023, April 6). The privacy considerations with something like ChatGPT cannot be overstated. In CNN. https://www.cnn.com/2023/04/06/tech/chatgpt-ai-privacy-concerns/index.html
- Mittelstadt, B. D., Allo, P., Taddeo, M., Wachter, S., & Floridi, L. (2016). The ethics of algorithms: Mapping the debate. Big Data & Society, 3 (2), 2053951716679679. https://doi.org/10.1177/2053951716679679
- National Institute of Standards and Technology (NIST). (2021). AI Risk Management Framework. Retrieved from https://www.nist.gov
- OWL.co. (2023). Bias in AI. Retrieved from OWL.co
- Selbst, A. D., & Barocas, S. (2018). The intuitive appeal of explainable machines. Fordham Law Review, 87 (3), 1085. Retrieved from https://ir.lawnet.fordham.edu
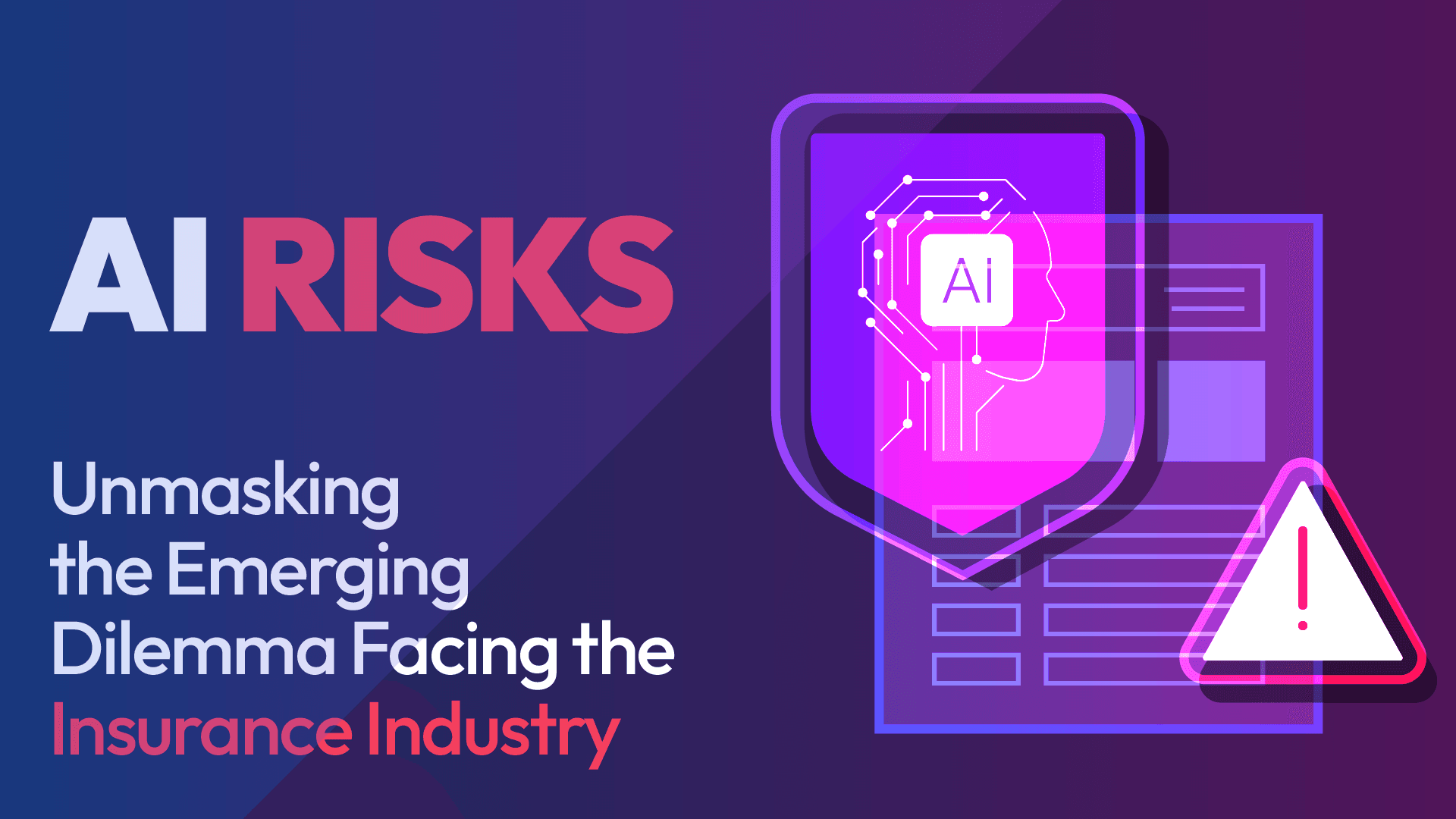