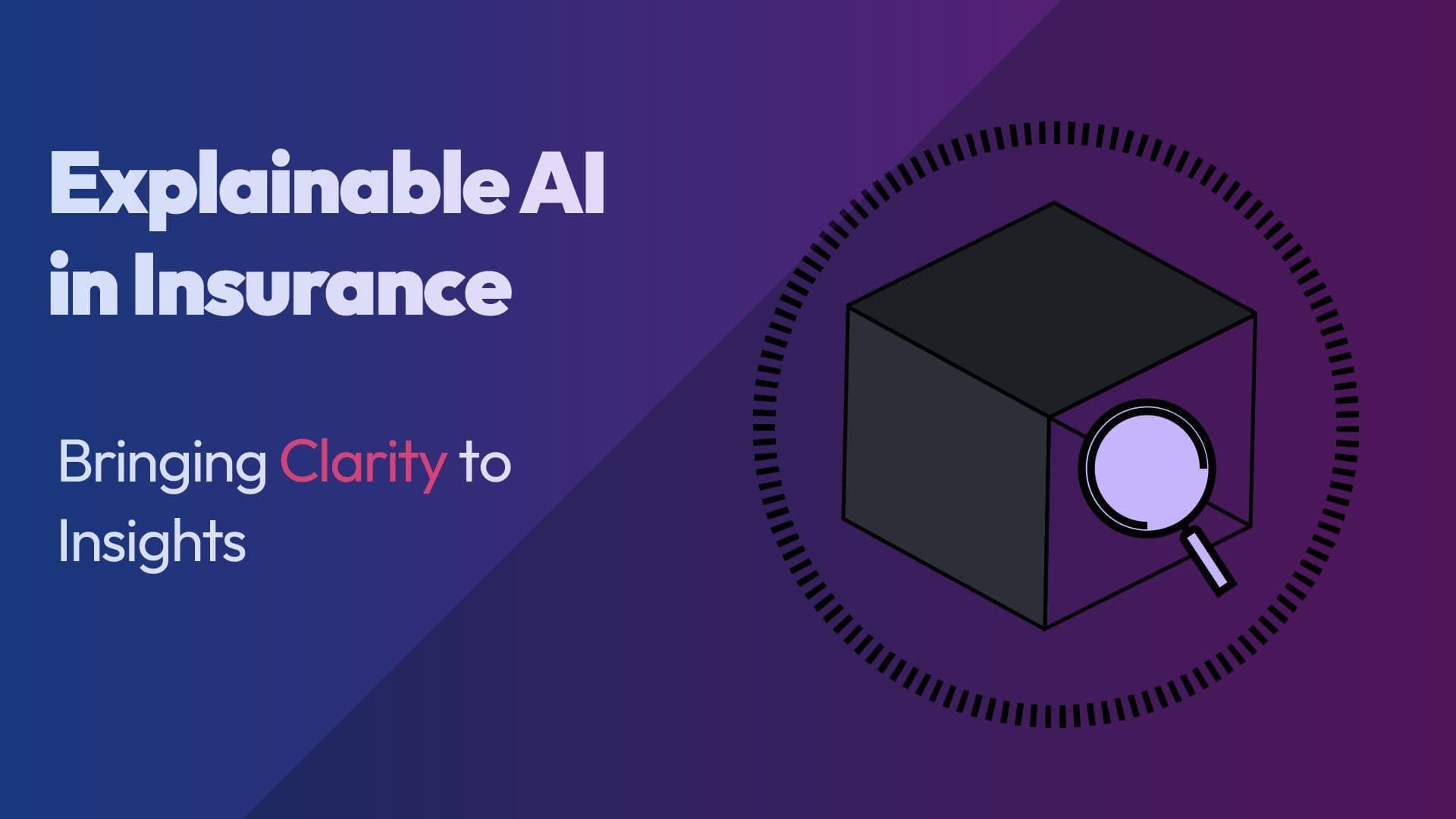
Explainable AI in Insurance: Bringing Clarity to Insights
Validate Insights with Explainable AI for Better Claims Decisions
Artificial intelligence is reshaping the insurance industry, empowering claims teams to drive better outcomes for insurers and claimants.
But as AI becomes more prevalent, the need for transparency grows.
If insurers can't trust the insights that AI generates, they'll struggle to demonstrate fairness, meet regulatory requirements, and uphold ethical standards in decision-making.
This is where explainable AI (XAI) comes in. Explainable AI provides clarity and transparency about its insights and recommendations, enabling claims & investigations teams to justify their decisions, stay compliant with regulations, and gain confidence in AI.
We'll outline the challenges of trust in AI, introduce explainable AI in insurance, and overview how explainable AI helps claims teams take control of their decisions to better support claimants and drive business growth.
The Challenges of Trust in AI for Insurance
When using AI in claims organizations, insurance companies can make more accurate decisions because AI provides insights for adjusters and investigators to better understand complex claims details.
AI bases its insights from data in medical files, legal documents, and more to help insurers assess claims, detect fraud, and evaluate risk.
But how can claims teams trust the veracity of these insights? Without knowing if the AI's insights are fully accurate, insurers risk higher litigation costs, more claims leakage, and compliance violations.
What Makes Trust Important for Insurance Companies
Trust ensures that claims teams can optimally improve outcomes, but there are multiple barriers to trust for insurance companies.
1. The "Black-Box Dilemma"
Some AI models act as "black boxes," generating outputs without explaining how the outputs work or came to be.
This lack of transparency makes it difficult for insurers to validate and justify AI-driven decisions.
For example, if AI flags a claim as potentially fraudulent and a handler thus denies the claim, the insurer will have to explain the decision to regulators, stakeholders, and customers. But explaining decisions is tough without understanding and trusting the AI's rationale.
Without clear knowledge, AI decisions can cause uncertainty, regulatory scrutiny, and reduced customer trust, undermining confidence in both the technology and the insurer.
2. Bias in AI
According to data, about 38% of all "facts" generated by AI contain bias.
This can happen when AI models are trained on historical data or influenced by biased past judgments, which can reinforce or amplify existing biases, leading to unintended discrimination, wrongful denials, or skewed risk assessments.
Artificial intelligence that explains the rationale behind its insights (remember "show your work" in high-school math class?) and enables claims teams to monitor & govern how the AI works is essential for mitigating bias and supporting claimants.
3. Regulatory Compliance, Privacy, and Security
With frameworks like GDPR, CCPA, and HIPAA, insurers face pressure to ensure AI is transparent, fair, and non-discriminatory.
Compliance requires adequately explaining and justifying AI-guided decisions at the risk of fines, legal trouble, reputational damage, and loss of trust.
4. Operational Oversight
AI for insurance relies on accurate and reliable outputs. But without proper oversight, teams risk overtrusting AI or overlooking inaccurate details in the claims insights the AI provides.
Explainable AI empowers claims teams to become accountable, oversee the AI's operations, and seize control of their decisions to ensure fair & transparent claims outcomes.
The Role of Explainable AI in Insurance
When frontline claims investigators can understand AI's insights, they gain autonomy over their decisions, reducing risk & litigation exposure, mitigating bias, and ultimately earning customer trust to provide better experiences—resulting in human-centric outcomes.
With full transparency via explainable AI, insurance companies can overcome the challenges of "black boxes," bias, regulatory compliance, and operational oversight.
But what is explainable AI, and how does it work?
What Is Explainable AI
Explainable AI enables human users to understand and trust the outputs created by AI. The processes of XAI are known as "explainability"—i.e., an AI model's ability to explain itself.
Explainability plays a critical role in ensuring AI-driven insights are transparent, interpretable, and justifiable. Unlike black-box systems that deliver outcomes without context, XAI provides clear, traceable views of AI's reasoning process.
Principles of Explainable AI
Explainable AI is all about building trust. It functions on some basic principles:
- Transparency: The AI is candid in justifying its outputs with explanations.
- Fidelity: The AI's explanations are contextually relevant and based on accurate data.
- Understandability: The AI's explanations are simple and easy to comprehend.
- Fairness: The AI's explanations demonstrate fairness towards all stakeholders.
- Traceable: The AI's explanations are rooted in citations that are easily discoverable.
When AI upholds these principles of explainability, users can trust the technology they're using to make informed, accurate decisions.
How Explainable AI Works in Insurance
Explainable AI in insurance is a set of processes that provide explanations for AI-generated insights about claims to insurance companies.
When insurers have clear explanations about claims insights from AI, they can validate those insights for accuracy, fairness, and reliability; justify their decisions to auditors, claimants, courts, and regulators; and proactively detect & mitigate biases in their decision-making.
Explainable AI vs. Accountable AI
Explainable AI is part of the concept of accountable AI for insurance—AI that empowers claims teams to act responsibly, best support their customers, and build trust & confidence.
AI is accountable when it's explainable, governable, and trained on relevant data. It must be able to delineate & justify its outputs; enable humans to retain control over its outputs; and base its outputs on data that's pertinent and bias-free.
For insurance, this means AI that:
- Describes the rationale behind its claims insights, including with citations—this is the explainability aspect;
- Allows claims teams to give feedback about insight accuracy so it can make proper adjustments; and
- Learns only from human feedback and relevant, past claims decisions, not historical data in general at the risk of making inaccurate predictions.
Explainable AI is a key component of accountable AI. When insurers leverage AI with explainability built in, it helps claims teams trust the AI, empowering them to make decisions with confidence and in turn gain the trust of customers.
Benefits of Explainable AI for Insurers
By providing clear views of its reasoning, XAI enables insurers to validate AI-generated outputs for accuracy, fairness, and reliability; offer clear, actionable explanations to auditors, regulators, and policyholders; and proactively detect & mitigate biases to support claimants.
These benefits play out in multiple ways, from promoting equity to making more informed claims decisions. Below are some specific examples of the strategic advantages of explainable AI for insurance companies:
- Establishing and sustaining trust in insurance decisions.
- Reducing bias and promoting fairness in outcomes.
- Ensuring compliance with regulatory standards.
- Improving fraud detection with greater accuracy.
1. Building Trust
Customers rely on insurers to deliver fair, accurate, and well-informed claims decisions. For insurers leveraging AI-generated insights, transparency and explainability are paramount.
Explainable AI justifies its own insights, reinforcing trust and confidence in the fairness and reliability of the decision-making process.
2. Mitigating Bias
AI carries the risk of inadvertently engendering inequitable outcomes. XAI provides insurers with tools to identify and address potential biases, which in turn help the AI improve over time, ensuring AI-driven processes align with ethical standards that insurers strive to live up to.
3. Staying Compliant
The increasing use of AI in insurance comes with heightened regulatory scrutiny. Insurers must demonstrate the rationale behind AI-driven decisions to meet evolving legal and compliance requirements.
Explainable AI facilitates compliance for insurance companies by offering clear and structured explanations for the logic behind AI-generated insights.
4. Preventing Fraud
The Coalition Against Insurance Fraud estimates that insurance fraud costs the U.S. about $308 billion annually. That's a lot of money! And insurance companies end up eating most of those expenses.
With explainable AI, insurers can better understand AI's reasoning for flagging claims as potentially fraudulent, reduce false positives, and enable their investigators to focus on substantiated patterns, enhancing the efficiency and fairness of their fraud detection.
Examples of Explainable AI in Insurance
Explainable AI plays a critical role in ensuring AI-driven insights are transparent, interpretable, and justifiable for insurance companies.
Here are some examples of explainable AI in insurance:
- AI that provides descriptive insights when it flags and ranks by priority potentially fraudulent claims;
- AI that links to sources from relevant claims documents when summarizing claims details;
- AI that outlines its rationale when answering user queries about a claim, including with citations.
For instance, OwlAssist from Owl.co helps insurance teams understand complex claims by answering user questions to simplify and summarize details. It's an example of explainable AI in insurance because it explains its rationale and lists its sources.
OwlAssist employs explainable AI by describing its reasoning when answering questions about claims details and linking to relevant claims documents as evidence.
Drive Human-Centric Claims Outcomes with Explainable AI
Explainable AI sets the foundation for a responsible approach to leveraging AI in insurance for equitable and informed claims outcomes.
Policyholders rely on insurers to make fair and well-reasoned decisions; insurers, in turn, must have confidence in the AI-driven insights guiding their operations.
By adopting Explainable AI, insurers can mitigate bias, reduce fraud, maintain regulatory compliance, and foster long-term customer trust.
And as an integral component of accountable AI, explainability forms a cornerstone of Claims Intelligence, knowledge that liberates claims teams based on AI that's accountable, effective, and ethical, so they can create a human-centric way forward for insurance.
Owl.co dedicates itself to empowering insurers with Claims Intelligence to drive this transformation. Book a demo to learn more about our AI toolkit for insurance.
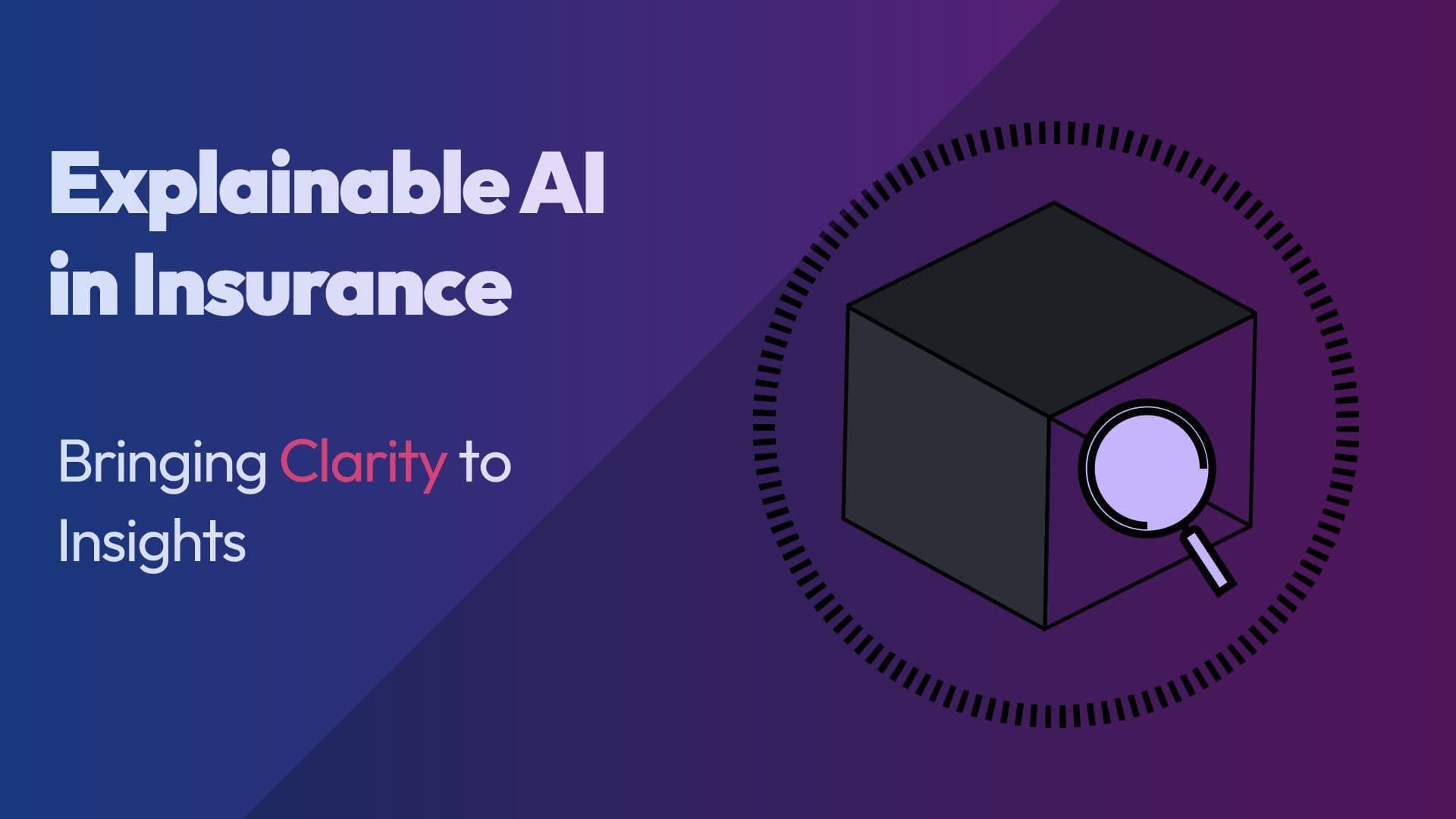