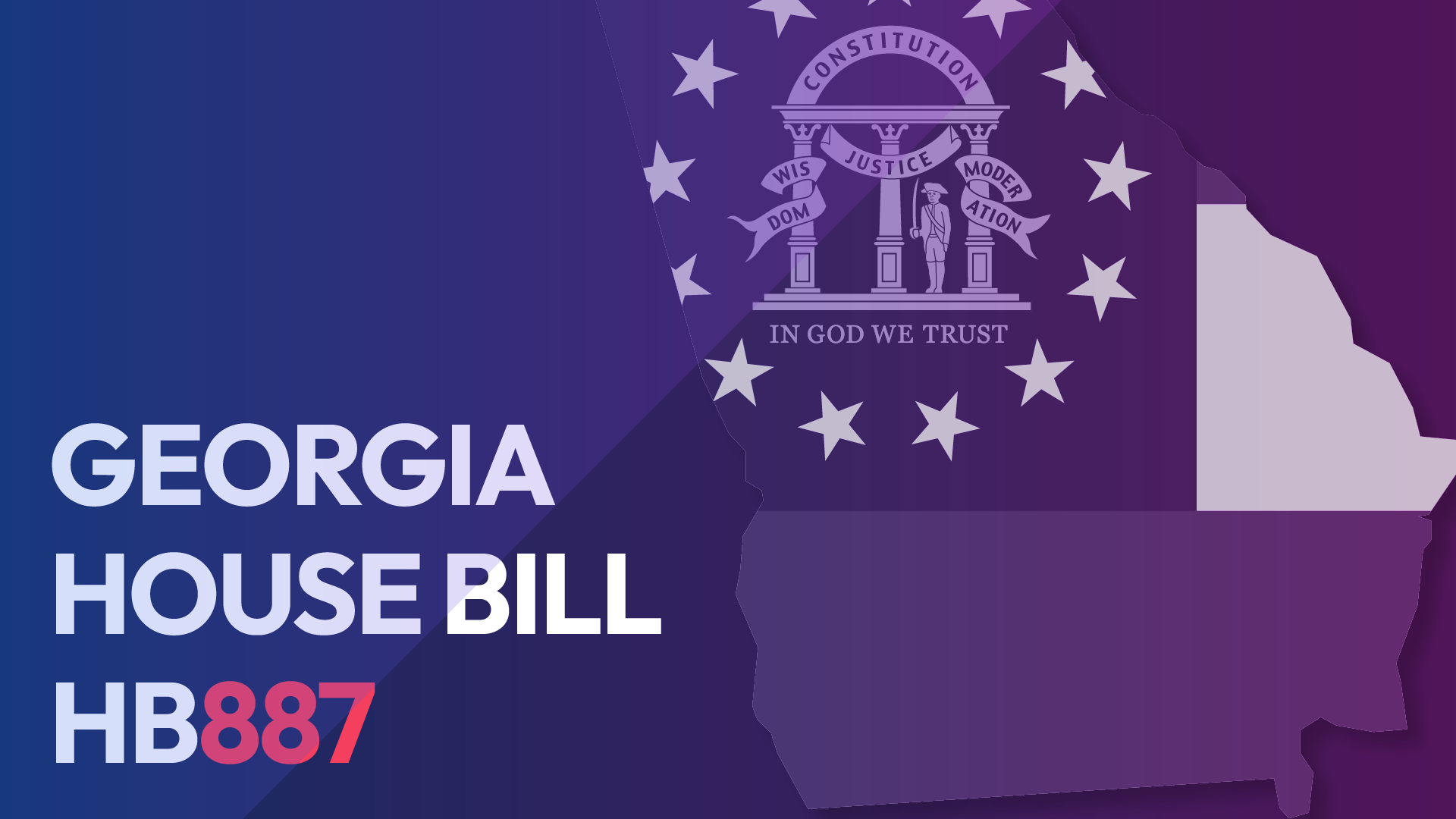
Understanding the Implications of the Georgia House Bill on Using AI in Insurance Decisions
TL;DR
- Georgia House Bill 887 aims to protect claimants from the inherent bias in predictive analytics based AI tools
- Descriptive or individualized analytics in AI avoids bias, since it does not make predictions based on historical data
- Owl.co is structurally different from its origin, relying on individualized analytics to provide claims insights
In this article, we'll explore Georgia House Bill 887 (HB887) and its implications for insurers. HB887 seeks to prohibit the use of artificial intelligence (AI) in making certain healthcare decisions, citing concerns about bias and discrimination. While these concerns are valid, it is important to recognize the potential benefits of AI in the insurance industry and the scope of AI in ultimately enhancing the decision accuracy and Net Promoter Score of an insurer.
We will delve into the key provisions of HB887 and examine the downside of using predictive analytics in insurance decisions, which is what HB887 is intended to safeguard against. We will also explore descriptive analytics as an ethical and effective alternative, and discuss how insurance companies can still leverage AI in claims processing and decision-making.
Understanding the bill's key provisions and what it means to insurers
Georgia House Bill 887 (HB 887) has generated significant attention in the insurance industry due to its implications for the use of artificial intelligence (AI) in healthcare decisions. This bill seeks to address concerns about bias and discrimination in AI-driven decision-making processes. Understanding the key provisions of HB 887 is crucial for insurers seeking to comply with the proposed regulations and continue leveraging AI responsibly.
At its core, HB 887 aims to prohibit the sole reliance on AI or automated decision tools in making healthcare-related decisions. Insurers must establish procedures for meaningful review of any coverage determination resulting from AI or automated decision tools by an individual with the authority to override such tools. This provision makes sure that AI-driven decisions are subject to human oversight and intervention, mitigating the risk of biased or erroneous outcomes.
HB887 is not and will not be the only one of its kind. With the increasing adoption of AI in business, healthcare and insurance, the question of ethical use of technology is an appropriate and important one.
The bill uses the term AI in its widest sense, but not all AI is the same. There is however, potential danger in using some AI and machine learning methods to make insurance decisions, as we will explore in the next section.
The shortcomings of predictive analytics in the insurance industry
One of the biggest concerns about predictive analytics is that it can lead to biased and discriminatory practices. This is because predictive models are often trained on historical data, which can reflect existing biases and prejudices. For example, if a predictive model is trained on data that shows that women are more likely to file claims for certain types of illnesses, then the model may predict that women are more likely to be high-risk customers. This could lead to women being charged higher premiums or denied coverage altogether.
Another concern about predictive analytics is that it can perpetuate existing inequalities. For example, if a predictive model is used to determine which customers are eligible for discounts or rewards, then it is likely to benefit those who are already well-off. This is because these customers are more likely to have the data that the model is looking for, such as a good credit score or a history of safe driving. There is also a lack of transparency and accountability in predictive models. It can be difficult to understand how predictive models work and why they make certain decisions. This can make it difficult for customers to challenge decisions that they believe are unfair or inaccurate.
Finally, predictive analytics can lead to a lack of human oversight and control. This is because predictive models can sometimes make decisions without any human input. This can be dangerous, as it can lead to mistakes being made that could have serious consequences for customers.
Let's consider an ethical alternative to using AI in insurance claims decision - individualized analytics.
What is individualized analytics and how is it different from predictive analytics
Individualized analytics refers to the practice of analyzing and interpreting data at an individual level to gain insights and make informed decisions. It involves going beyond generalized models and historical data to understand the unique characteristics, circumstances, and behaviors of each individual. This approach is particularly valuable in industries like insurance, where personalized recommendations and tailored strategies can significantly enhance outcomes.
One of the key advantages of individualized analytics is its ability to provide enhanced accuracy and precision. By considering specific attributes and data points related to an individual, such as their medical history, employment history, and online activities, professionals can make more accurate assessments and recommendations. This level of granularity allows for a deeper understanding of each individual's needs and circumstances, leading to more precise and effective decision-making.
Furthermore, individualized analytics can greatly improve the customer experience. By engaging with individuals on a personal level and offering solutions that resonate with their specific circumstances, insurance providers can foster trust, loyalty, and satisfaction. This personalized approach demonstrates a genuine understanding of the individual's needs and can lead to higher customer retention rates.
Another significant benefit of individualized analytics is the mitigation of bias and discrimination. Unlike predictive models that may perpetuate biases present in historical data, individualized analytics prioritize fairness and equity by focusing on the unique characteristics and circumstances of each individual. This helps ensure that decisions are made objectively and without bias, leading to a more fair outcome for all parties involved.
How is Owl.co different
Since its founding in 2018, Owl.co has stood for the ethical use of AI in insurance claims handling. When it comes to claims, each file is different and unique to an individual, with nuances that can significantly influence the decision outcomes.
Traditional AI claims management tools would create ‘claimant profiles’ that are both based on biased machine learning inputs and promote cognitive bias by indicating to adjusters that a certain candidate is likely ineligible. Owl.co’s individualized analytics methods to use Natural Language Process (NLP) and Large Language Model (LLM) to analyze each claim file, independent of other files to surface deep and hidden insights and trends of the claimant.
In addition to bias being removed from the system, Owl.co’s platform offers transparency to the decision made by the claims adjuster, by highlighting data from the claim file.
Unlike predictive AI tools that offer decisions even when there is insufficient data, Owl.co enriches claim files with external publicly available data to enhance decision accuracy.
Contact our team to learn more about our approach to claims handling or get a live demo of the platform.
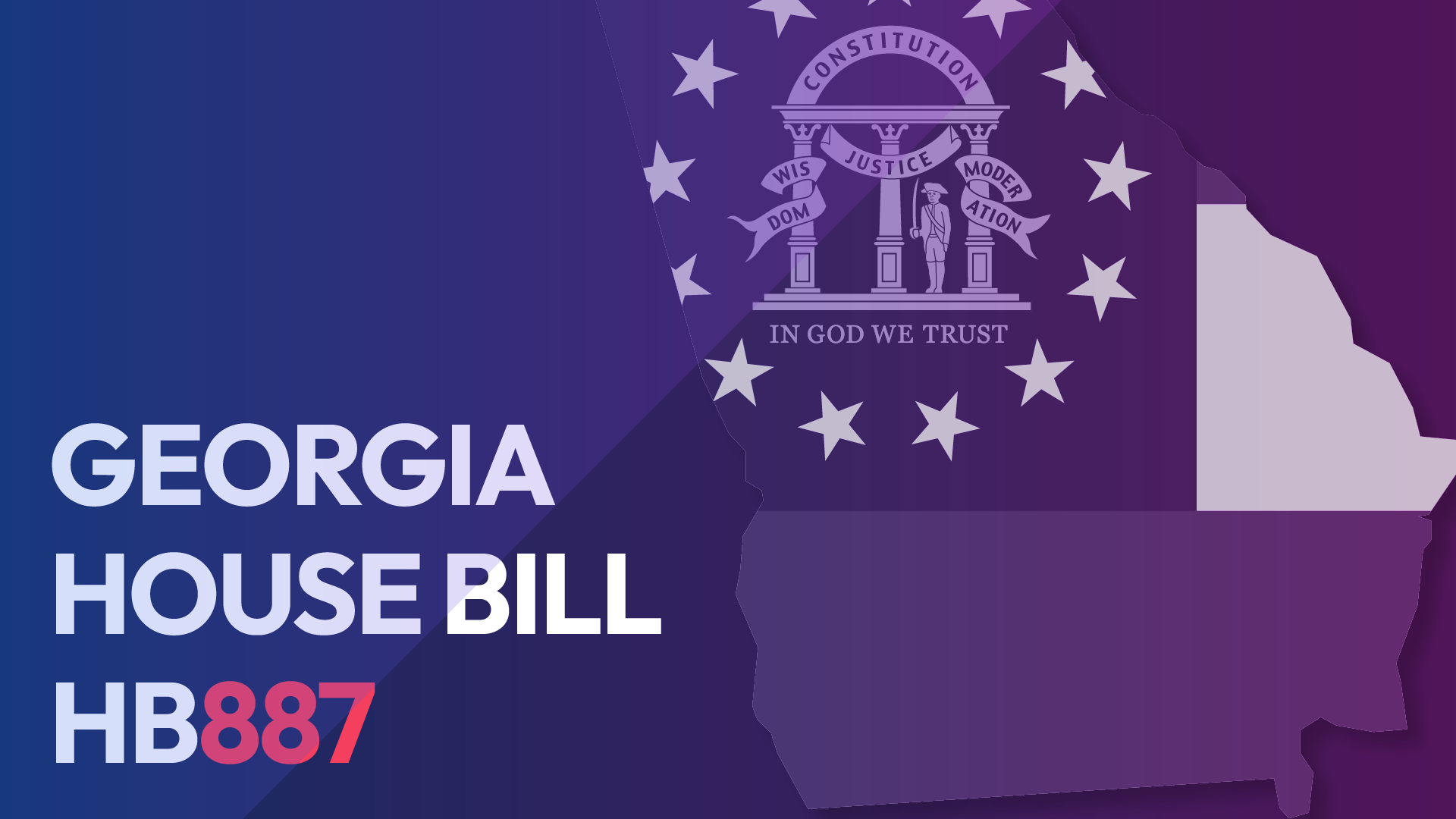